Dr. Mark Fuge will be promoted to Associate Professor with tenure at the University of Maryland College Park, effective later this summer. Congratulations, Mark!
Mark Fuge graduated with a Ph.D. from UC Berkeley in 2014 with dissertation: Collaborative Design Informatics: Leveraging Big Data to Create Better Designs.
As an Assistant Professor of Mechanical Engineering at the University of Maryland, College Park he started a research program in Design Informatics/ Data-driven design at the intersection of Mechanical Engineering, Machine Learning, and Design. This research builds on his work in applied machine learning, optimization, network analysis, additive manufacturing, human-computer interfaces, crowdsourcing, and creativity support tools.
Mark has received many awards as an assistant professor. Mark was awarded a DARPA Young Faculty Award in 2017 for a project entitled “Topology and Synthesis of Design Manifolds” as part of the “Functional Mathematical Tools for Design” topic area. The grant builds upon our past work in Design Manifolds by studying how multiple part manifolds interact in assemblies and how mathematical tools from topology, group theory, and machine learning can aid human exploration of large, complex design spaces.
Mark won the NSF CAREER Award in 2020 on “Learning Design Representations: The Effect of Differential Geometric Manifolds on the Inference of Design Structure.” Here is uses Engineering Design and Machine Learning to enable computers that learn how to design complex systems like new aircraft or vehicles.
Within Engineering Research, Dr. Mark Fuge is a rising leader in the frontiers of engineering in the area of Machine Learning for Engineering Design. Specifically, he has advanced this emerging frontier in several ways:
- He has published numerous papers in the top journals of his field on using Machine Learning (ML) techniques to discover mathematical representations of engineered systems useful for design and optimization. For example, he has published a growing body of work on low-dimensional subspaces called Design Manifolds that describe engineered components like airfoils, hierarchical assemblies of parts, and components that obey mathematical symmetries like rotational symmetries or permutation symmetries. These results have led to advances in optimization and design, for example, by reducing the computational cost of aerodynamic optimization by an order of magnitude.
- In the process of defining this emerging frontier of engineering research, he has also made scientific contributions to related fields like Machine Learning by publishing in top venues in Computer Science including, ACM’s Computer-Supported Cooperative Work, International Joint Conference on Artificial Intelligence (IJCAI), and the Neural Information Processing Systems (NeurIPS) Conference. This speaks to his ability to both advance his own field while creating concurrent advances in other fields and enabling communication between them.
- He has given invited talks on this new frontier of Machine Learning for Engineering Design at academic (Carnegie Mellon), industrial (United Technologies Research Center), and government (NIST, Army Research Lab, NASA Langley, NASA Goddard, ARPA-E) settings for those looking to leverage these techniques in engineering practice.
- He has helped lead special issues on this emerging topic for top Journals in the field, helping to push and support this emerging frontier. For example, he was the Guest Editor for the ongoing Special Issue on “Machine Learning in Engineering Design” for the Journal of Mechanical Design, the top journal within his sub-field and received the 2019 Guest Editor Award.
Mark has also taken a leadership role in a number of cutting edge research areas:
- He has partnered with researchers working in computational modeling of material science to apply Machine Learning to the design and optimization of new material systems for the Navy. While outside of his direct field, this speaks to Mark’s ability to translate advances in his own field to others, and fits within the spirit of the symposium.
- He has partnered with Roboticists and Clinical Medical professionals (Surgeons, Cardiologists) to implement 3D printable aortic grafts of surgery in children with congenital heart disease, leveraging his past work. This speaks to his ability to engage scientists outside of academic research (in this case clinical research) to translate emerging techniques to areas of social importance.
- He was one of four faculty selected university-side to attend the week-long Alan Alda Center for Communicating Science Bootcamp on how to communicate science and engineering’s value to a broader audiences including the general public, policy makers, and the press. This speaks to his ability to and willingness to engage with those outside of his field and make clear connections between his fundamental engineering research and others.
- He is presently the Chair of the Technical Committee for the Design Theory and Methodology division of the American Society of Mechanical Engineers (ASME). As such, he helps led and advocate for the technical advancement of Engineering Design within ASME.
- He has organized symposia on this emerging topic, bringing together researchers and thought leaders from across academia, government, and industry. For example, he hosted a symposium on Data-Driven Design at the University of Maryland in 2016 that included participants from NIST, Domestic and International University, the Navy, the National Science Foundation, and various industrial partners. It was the first symposium of its kind on this emerging topic, which has grown in size since then.
- On the theme of “Frontiers of Engineering”, he was awarded a workshop/ Summer School grant entitled “Emerging Mathematical Foundations of Engineering Design” to be held in Summer 2020. This demonstrates (1) willingness to bring together multiple fields (Math, CS, Statistics, and Engineering in my workshop’s case) as well as (2) evidence of helping push the frontiers of the field in new ways.
- His work has helped multiple federal agencies understand technical problems at this emerging frontier and help them craft funding programs to address fundamental scientific and engineering challenges around using computers to generate and learn from the design of engineered systems. This is evidenced by new federal funding programs such as DARPA’s Fundamentals of Design program, and ARPA-E’s Machine Learning Enabled Energy Product Development programs that used his work, in part, as the basis for justifying their federal programs and grow this emerging field. This speaks to his ability to lead and grow the community of researchers interested in and capable of solving these problems through advocating and developing federal support for this emerging area. He has secured $10M in grant funding for expanding the intersection of Engineering Design and Machine Learning.
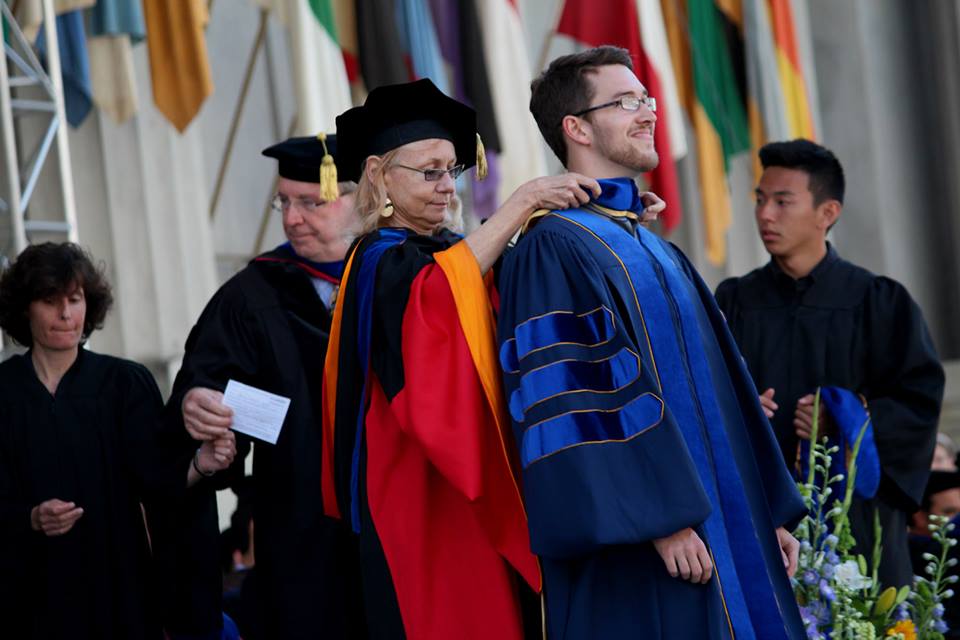
Mark Fuge graduated with a Ph.D. from UC Berkeley in 2014 with dissertation: Collaborative Design Informatics: Leveraging Big Data to Create Better Designs.
Top feature caption from University of Maryland: Meet the Makers.